Faculty Profile
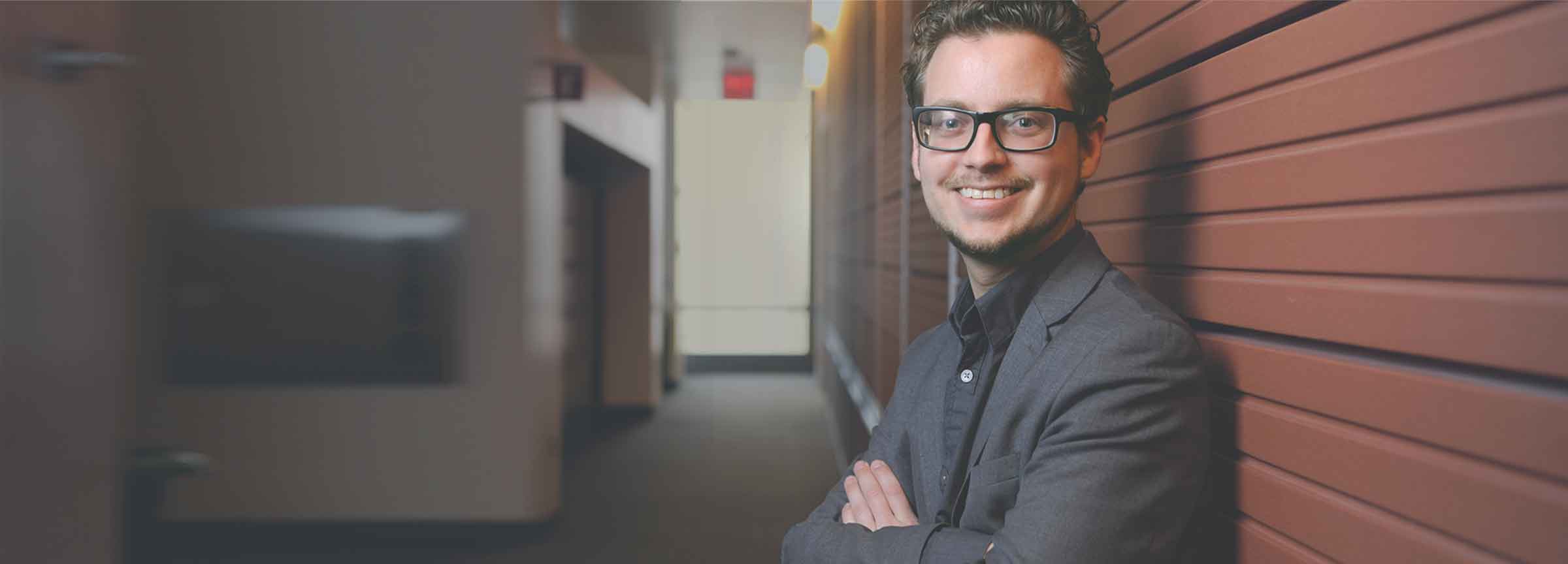
John Chodera
The focus of our laboratory is building quantitative, predictive models that allow us to rationally design new small molecules either as tools for chemical biology or potential new therapeutics, with a special emphasis on designing selective kinase inhibitors, the next generation of nontoxic but effective therapies against diseases like cancer.
A key part of our approach is the use of large numbers of consumer-grade graphic processors, both in local computer clusters and in the Folding@home distributed computing project founded by my postdoctoral advisor Vijay Pande at Stanford, where hundreds of thousands of people around the world donate their computer cycles to help biological research. Folding@home donors are excited to be engaged in the science as well as contribute to massive studies that would be otherwise impossible; they effectively donate electricity and hardware to research in human health and disease, often upgrading their systems simply to participate in these large-scale research projects. It is a fantastic resource that has produced over a hundred scientific publications, as well as huge data sets for researchers to mine. It boosts the kinds of computations we can do with the same amount of funding by orders of magnitude, so that is a fantastic resource.
We couple these computational approaches with advances in both theory and automated biophysical experiments. We spend much of our time testing models in the laboratory, making a multitude of predictions with computation, then make perturbations to biomolecular targets through engineering in mutations in order to make measurements that validate and refute our models. We actually learn a lot from failure, so our laboratory is set up to fail cheaply and quickly over and over again in order to drive the iterative improvement of our computational models. We’re able to do this without losing our minds by relying heavily on robotics, automating experiments in our wetlab to make predictions about how mutations will lead to differences in drug binding affinities. In our case, we essentially invert the drug discovery problem to make this process rapid and inexpensive – instead of designing new small molecules and synthesizing them, we can make and test predictions more cheaply and with higher throughput by mutating the biomolecular target and assaying the affinity to a library of small molecules.
We take the kinases or the biological molecules that are the targets for these therapeutics, buy a bunch of small molecule therapeutics, and then mutate the protein by introducing point mutantations. Physics doesn’t care which binding partner we change — we can modify either the protein or the small molecule to test predictions in how these modifications will change affinity. In our case, the robots are very good at engineering many small changes to the protein in parallel, where we attempt to predict which changes will have the most impact or tell us the most about how to improve our models. We then make those mutants in parallel, assay them against the wide variety of drugs, and then learn from the data that we do and do not understand about how to improve our models, where the next generation of predictive computational models will help us better rationally design new small molecules. We can also use this platform to study one of the more important ways in which cancer therapies fail — the emergence of resistance. We want to understand how resistant mutations arise, as well as how we can design new therapies that are less likely to elicit resistance, or at least get a leg up on designing the next generation of therapeutics to overcome the resistance we expect to see.
The interdisciplinary nature of the CBM program makes it possible to tackle challenging problems in human health and disease using any method, any methodology, any discipline, any instrumentation that can possibly help us make progress. In my own laboratory, we have a mechanical engineer, an electrical engineer, a toxicologist, a biochemist, a biophysicist, and a physicist. Bringing all of this expertise together allows us to make inroads into traditionally difficult problems. Beyond my own laboratory, as I look out the window of my office, there are at least a thousand faculty just on this one street corner, all working on important problems in human health and disease; the density of intellect here is simply unmatched.